COVID-19 Testing – What’s Sensitivity and Specificity, and how helpful is it in population screening?
COVID-19 Testing – What’s Sensitivity and Specificity, and how helpful is it in population screening?
Medicine is an evolving profession, and it has come a long way since ancient physicians relied on observation and their ability to perform limited examinations. A notable development in medicine was introducing ”the laboratory” at the beginning of the 20th Century. With this came increasingly sophisticated tools and techniques, giving today’s modern physicians an array of diagnostic tests. However, a clinically helpful test must provide reliable information influenced by both analytical and non-analytical factors.
Analytical factors include the sensitivity and specificity of the test, which are used in the media due to COVID-19 reporting. These terms refer to the test’s ability to reliably predict whether an individual does or does not have the viral infection causing COVID-19. These are mathematical expressions of the test’s false-positive and false-negative rates. A test with high sensitivity correctly identifies a high percentage of individuals who have the virus, and a high specificity will correctly identify a high percentage of individuals who do not have the virus. For example, if we test 100 people who have the virus with a test of 99% sensitivity, we will identify 99 out of those 100 individuals (1 false-negative). In the case of specificity, if we test 100 people who do not have the virus, the test will correctly identify 99 people as negative (1 false-positive).
However, we do not always know the actual infection rates of populations across the globe – after all, this is why we want to perform the tests. Therefore, if we apply the standard sensitivity and sensitivity rules to a population with an estimated relatively low prevalence of infection, the false positives will soon outnumber the false negatives and give less meaning to the test results. So how do we apply these values so they have real sense across real-world populations? This is where some sophisticated statistics are put in place. The sensitivity and specificity rates are used to calculate predictive values, which inform us how valuable the test would be when applied to a population, i.e. the probability of correctly identifying a viral infection. The upshot is that the simple statistics reported for tests do not reflect the potential prediction rates in populations which is essential for evaluating if a particular test is useful.
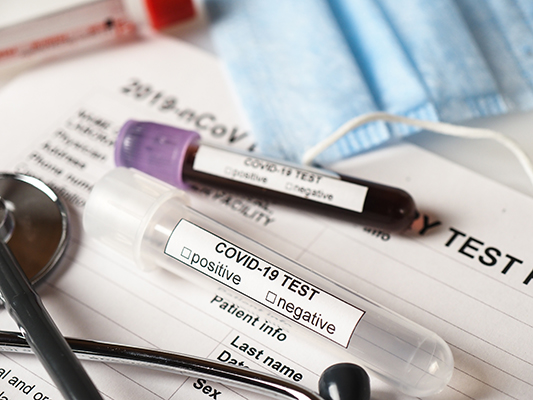